Table of Contents
- Introduction
- Mediating Transformation of AI in Peer Review
- Balancing Innovation with Integrity
- Navigating Challenges and Risks of AI in Peer Review
- Charting the Future of AI in Peer Review
- Conclusion
Introduction
Artificial intelligence (AI) has become ubiquitous in various fields. The article explores AI in peer review, covering its potential use and benefits.
Peer review has played a pivotal role in upholding the integrity and quality of academic research for decades. Before research is published, it undergoes rigorous evaluation by experts in the field, who scrutinize the methodology, analysis, and claims made in the study. This peer review process is essential for identifying flaws, limiting the influence of biases, and ensuring only high-quality research sees the light of day.
However, peer review has its challenges and limitations. It can be highly subjective, inconsistent across reviewers, and painfully slow. This is where AI comes in—with the potential to enhance efficiency, consistency, and accuracy in peer review on an unprecedented scale. From automated screening of manuscripts to algorithms detecting statistical and logical errors, AI tools are bringing about a renaissance in scholarly evaluation.
Peer review dates back over 300 years, with the first peer-reviewed scientific journal, Philosophical Transactions of the Royal Society, established in 1665. Since then, pre-publication peer review has become the cornerstone for upholding rigor and integrity in scholarly communication across scientific disciplines.
By leveraging the expertise of independent researchers in a specific field, peer review aims to identify methodological flaws, ethical issues, factual inaccuracies, and other problems before the research gets published. This guards against disseminating invalid or poor-quality studies that could undermine scientific progress.
Robust peer review is gatekeeping in academic publishing—screening out unfounded claims, conflicts of interest, and questionable research practices. It also helps improve manuscripts through constructive feedback to the authors. These characteristics have cemented peer review’s significance in safeguarding research quality.
The growing application of AI in publishing is transforming peer review in unprecedented ways—enhanced efficiency, consistency, accountability, and transparency represent some of the key areas where AI is bringing about positive change.
Automated tools now help screen submissions, check statistics, detect plagiarism and manipulation, and summarize reviewer feedback. This expands the bandwidth of human editors and reviewers. AI also assists in reviewer discovery and selection, helping editors find the best-qualified experts faster.
Such innovations promise to accelerate scholarly communication while also strengthening integrity policies. With continuous advances in AI, peer review appears poised for a renaissance that balances productivity and rigor in scientific evaluation.
However, as with any new technology, AI raises fresh concerns regarding transparency, bias, and ethical oversight. Realizing the transformational potential of AI in peer review without compromising the core principles of scholarly integrity remains an open challenge.
Mediating Transformation of AI in Peer Review
Morressier is a platform that utilizes AI and machine learning to streamline the peer review process. Their system automatically checks submitted manuscripts for plagiarism, readability, grammar, and more. This allows editors and reviewers to focus their efforts on assessing the quality and originality of the research rather than catching basic errors.
Enago has incorporated AI tools to improve the objectivity and consistency of peer review evaluations. Their system scans submissions and highlights sections that may require further scrutiny for potential manipulation or falsification of data. This aids reviewers in making informed decisions and ensures research integrity.
AI has introduced significant efficiencies in the peer review workflow. Natural language processing techniques can extract key information from manuscript submissions to auto-assign reviewers. This reduces administrative workload and speeds up the process of finding qualified reviewers.
Automated systems can also analyze reviewer comments and scores to provide editors with an assessment of a manuscript’s overall quality and areas needing improvement. Rather than manually combing through reviews, editors can utilize these AI insights to guide their decision-making.
AI has cut review and decision turnaround times nearly in half at some journals by accelerating initial checks and extracting key insights from reviews. This allows authors to revise and resubmit their work faster, increasing productivity and innovation across scholarly publishing.
Balancing Innovation with Integrity
As AI technologies become increasingly integrated into peer review systems, they must uphold the standards of transparency, objectivity, and accountability that are essential to preserving research integrity. While AI promises enhanced efficiency and accuracy, we must be mindful of potential unintended consequences and risks.
Upholding research integrity
AI systems should be carefully designed and validated to reduce biases and ensure fair, ethical decision-making. Ongoing auditing of algorithms by independent third parties can identify issues early. AI should also provide explanations for its judgments to maintain interpretability and trust.
High-quality training data, representing a diversity of perspectives and avoiding skewed data, is key to mitigating unfairness. AI systems must be flexible enough to correct errors and incorporate new information and social norms as they evolve.
Ethical considerations
We must establish oversight frameworks, such as ethics boards and mechanisms for redress, to monitor AI systems in peer review and address issues as they arise. Researchers have proposed tools like algorithmic impact assessments to identify potential harms systematically.
AI should not fully replace human judgment in peer review but act as a supportive tool while editors and reviewers make ultimate decisions. This allows us to leverage the strengths of both human and machine intelligence.
Navigating Challenges and Risks of AI in Peer Review
As AI systems are increasingly integrated into the peer review process, concerns around algorithmic bias have come to the forefront. Machine learning models can inadvertently perpetuate or amplify societal biases if the training data contains uneven representations across different demographics.
For example, an AI system trained primarily on submissions from male researchers may rate papers from female scholars lower. Maintaining fairness and inclusivity is vital for peer review to fulfill its purpose of upholding research integrity. Biases creeping in could systematically disadvantage underrepresented groups, exacerbating existing inequities in academia.
Proactive steps are needed to ensure AI systems account for diversity and make evaluations based solely on the research quality rather than the authors’ identity. Periodic audits, diverse training data, and external oversight represent ways to promote algorithmic accountability.
Transparency and interpretability
As peer review leverages more AI, it becomes imperative that these intelligent systems remain transparent and interpretable. The reasoning behind decisions made by AI tools should be explainable to editors and researchers to build trust. For instance, highlighting the key passages, data, or factors influencing a particular recommendation allows for meaningful human oversight.
Complete opacity around how AI models arrive at conclusions threatens the credibility of digitally transformed peer review. Researchers are unlikely to accept evaluations without understanding the underlying logic. By prioritizing interpretability through attention mechanisms, modular designs, and interactive visualizations, publishers can uphold peer review’s reputation as a rigorous and objective process even in an AI-powered future.
Charting the Future of AI in Peer Review
As AI continues to transform peer review, it is essential to consider perspectives from experts in the field. Navigating the promises and perils of AI in peer review requires nuanced discussion and level-headed policies. We must strike a careful balance between realizing efficiency gains from automation while upholding principles of accountability and transparency.
One approach is developing AI tools with “human-in-the-loop” oversight at critical decision points. Hybrid review models would leverage AI assessments to assist human editors and reviewers. This allows AI to handle routine tasks like initial manuscript screening while reserving human judgment for complex evaluations. Relatedly, AI systems must be interpretable and clearly explain suggested decisions. Making algorithms more transparent without compromising IP promotes trust in the impartiality of their recommendations.
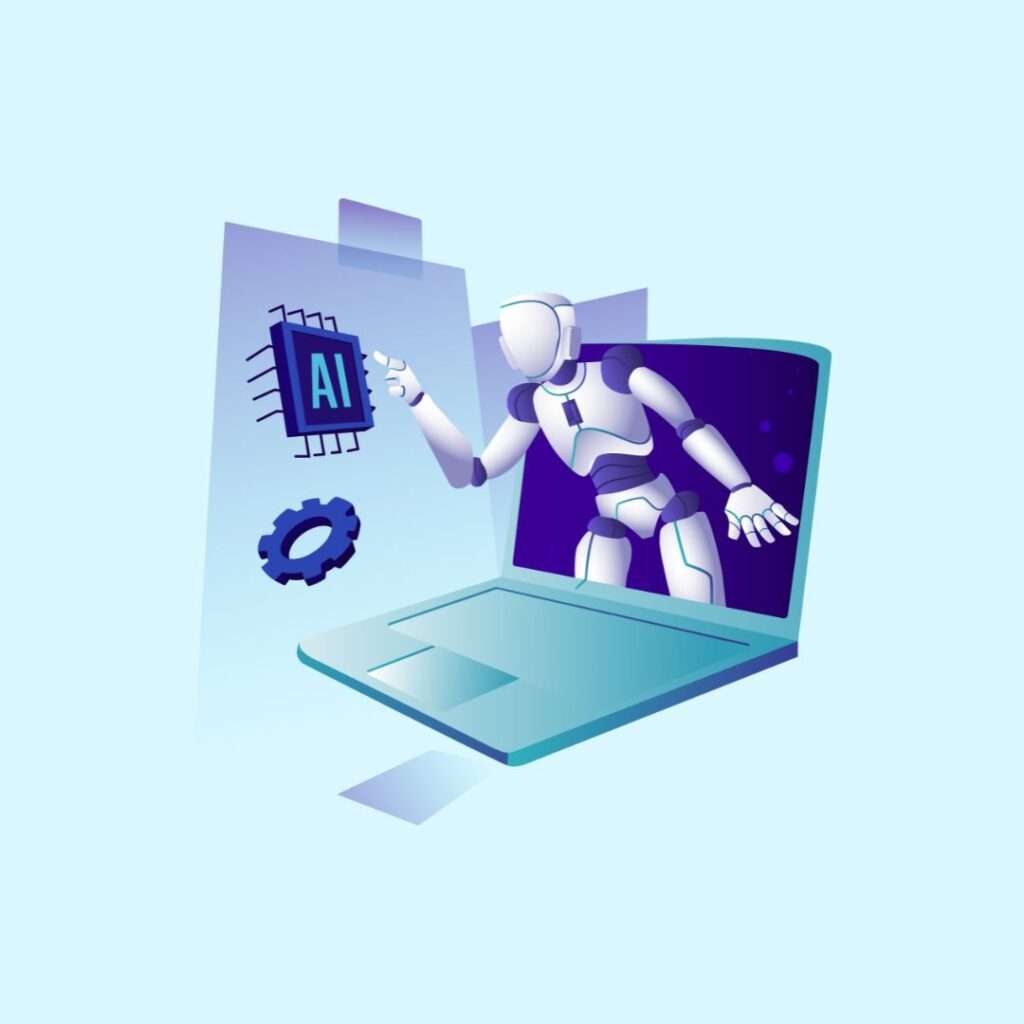
Overall, the goal should be responsibly expanding the frontier of innovation in scholarly communication through AI while grounding deployments in ethical frameworks that honor long-held peer review values. With conscientious effort on all sides, an AI-mediated renaissance in peer review can come to fruition.
Conclusion
As we have seen, artificial intelligence catalyzes a renaissance in peer review. AI tools streamline workflows, enhance efficiency, and improve evaluations’ accuracy. At the same time, these technologies introduce risks around issues like algorithmic bias. Maintaining integrity in peer review requires balancing innovation with ethical oversight.
In summary, here are some of the key takeaways on the impact of AI on peer review:
- AI is transforming peer review by automating routine tasks, detecting errors, checking for plagiarism, and more.
- These innovations have the potential to greatly accelerate and enhance scholarly evaluation.
- However, biases can be inadvertently baked into AI systems, threatening fairness.
- Transparency and interpretability are essential – “black box” systems undermine trust.
- Responsible implementation of AI requires balancing productivity with principles.
Empowering Responsible Innovation
A proactive, ethical approach is needed to harness the potential of AI in peer review while safeguarding research integrity. Some recommendations include:
- Developing codes of conduct and best practices for using AI in peer review.
- Instituting ethics review boards to assess algorithms before implementation.
- Fostering diversity in the teams designing these AI systems.
- Building interpretability directly into algorithms.
- Auditing systems continuously to identify issues like bias.
With deliberate effort, AI-powered innovation in peer review can flourish responsibly – upholding the principles of transparency, fairness, and accountability that underpin research integrity.