Table of Contents
- Introduction
- Understanding Semantic
- The Evolution of Semantic AI in Academia
- The Future of Semantic AI in Academia
- Conclusion – Embracing the Intersection of Semantic AI in Academia
Introduction
The write-up discusses Semantic AI in academia. Semantic AI is an emerging field of artificial intelligence focused on understanding the meaning of language. Unlike traditional AI approaches that analyze statistical patterns in data, semantic AI aims to comprehend the more profound significance behind words and how concepts relate.
Semantic AI involves creating mathematical representations of word and sentence meanings using techniques like natural language processing. This allows semantic systems to interpret more complex ideas expressed through language and even answer questions based on that understanding.
Critical capabilities of Semantic AI include:
- Analyzing the context and relationships between words/concepts
- Translating between languages while preserving meaning
- Answering questions based on comprehension of content
- Summarizing large volumes of text by identifying key ideas
Unlike keyword matching, Semantic AI aims to unlock deeper meaning within language. This could greatly expand the potential of AI across diverse fields.
The Growing Role of AI in Academic Research
Within academia, AI is taking on an increasingly vital role in knowledge discovery and dissemination. Semantic AI explicitly brings new opportunities to enhance scholarly communication and research.
A few applications include:
- Semantic search – Finding relevant studies/data based on meaning, not just keywords
- Automated meta-analyses – Systematically reviewing bodies of literature to uncover connections
- Intelligent research assistants – Answering researcher questions by comprehending background literature
Semantic AI could allow more impactful discoveries as research becomes more data-intensive and cross-disciplinary. Exciting times may lie ahead at the intersection of human creativity and machine understanding.
Understanding Semantic
Semantic, in short, means a study of meaning. Semantic understanding refers to comprehending the meanings and relations of words and sentences in their context. This comprehension extends beyond identifying keywords to grasping nuances, intentions, connotations, and implications conveyed through language. It involves recognizing the different meanings that a word or phrase may have depending on the situation and how combinations of words can generate complex ideas and concepts.
To unpack how AI, particularly Semantic AI, could impact or change our grasp of semantics, we must consider the evolution of language models and their ability to parse and generate language with increasing sophistication. Early AI systems relied on keywords and statistical patterns to interpret text; however, they lacked a genuine understanding of meaning and context. The gap between human-like semantic understanding and machine interpretation has been a substantial challenge for AI researchers.
There has been a significant shift towards models that can internalize more intricate language structures and meanings in recent years. This is primarily due to improvements in natural language processing (NLP) techniques, such as developing deep learning models that can create embeddings—vector representations of words—that capture semantic relationships. These advancements allow AI to discern context and effectively learn from vast amounts of textual data, enabling it to understand and manipulate language in ways that seem more ‘human.’
Semantic AI operates by using these sophisticated models to address tasks that require deeper language understanding, such as:
- Analyzing the context in which words are used to appreciate their specific meanings in that context.
- Translating between languages while maintaining semantic integrity involves understanding idiomatic expressions and subtleties in both the source and target languages.
- Answering questions not by retrieving pre-stored answers but by interpreting information in a given corpus or dataset and generating an informed answer.
- Summarizing content by recognizing central themes and arguments in a text through understanding the value and significance of different passages or data points.
The change brought about by Semantic AI in academia can influence how information is curated, analyzed, and synthesized. AI systems enabled with semantic capabilities can assist researchers in sifting through massive datasets and literature by locating relevant information based on the interconnected meanings of concepts rather than surface-level keywords.
This can revolutionize meta-analyses, which aim to combine the findings from multiple studies into a coherent understanding of a field or phenomenon. Furthermore, these systems offer the potential to act as intelligent research assistants by providing succinct summaries, identifying gaps in knowledge, suggesting related topics of interest, and even predicting future research trends.
These AI-driven semantic systems will likely change academia by making research processes more efficient and enabling the handling of more complex and nuanced inquiries. They might also facilitate cross-disciplinary research by bridging the semantic gaps between knowledge domains.
As more advanced models are developed, the potential applications of Semantic AI in academia will likely expand, continuing to transform how knowledge is discovered, shared, and built upon.
The Evolution of Semantic AI in Academia
AI’s deep learning capabilities revolutionize academic publishing by enabling Semantic AI systems to decode the meanings hidden within complex research papers and datasets. Powerful deep learning algorithms can now analyze millions of academic documents, interpret results, and identify critical insights faster and more accurately than humans alone.
Whereas traditional techniques rely on rules-based analysis, deep learning algorithms utilize neural networks that continually learn from vast datasets. Semantic AI can better understand academic language, terminology, and context when interpreting research findings.
Semantic AI systems can revolutionize how discoveries are presented and accessed by decoding the underlying semantics. Research can be automatically summarized, connected across disciplines, and translated into plain language for broader reach.
Semantic AI can conduct predictive analytics to forecast emerging research topics and trends by leveraging its deep comprehension of scholarly content. This allows publishers and institutions to make data-driven decisions about research directions and resource allocation.
Researchers also benefit from Semantic AI, identifying papers and authors to collaborate with, surfacing funding opportunities, and anticipating future citations and impact metrics for their work.
The peer review process is vital for upholding academic quality and integrity. Unfortunately, issues like bias and inconsistency often undermine its objectivity. Semantic AI promises to mitigate these concerns and improve peer review.
By assessing the semantics of research rather than surface qualities, Semantic AI reduces human propensities to bias. It also applies consistent standards when evaluating submissions. Together, this enhances fairness, accountability, and transparency.
Many publishers now use Semantic AI in academia to screen papers before assigning reviewers. This lightens reviewer workloads while allowing them to focus on higher-order assessments of merit and significance.
The Future of Semantic AI in Academia
The future of Semantic AI in academia appears promising and multidimensional, shaping research methods and the dissemination and evaluation of academic work.
Research Methodology Enhancement
Semantic AI is poised to further enhance research methodologies by facilitating nuanced text analysis and data interpretation. Comprehending complex academic language will enhance researchers’ capabilities to sift through large volumes of literature, extract relevant information, and discern patterns in data that might otherwise go unnoticed. This will be particularly important in fields that are burgeoning with new information, such as genomics and climate science.
Interdisciplinary Bridges
The application of Semantic AI could also help bridge disciplinary silos in academia. By recognizing and linking related concepts across disparate fields, Semantic AI systems might suggest novel research angles or unexplored collaborations that could lead to innovative breakthroughs. Overcoming the traditional barriers between disciplines can foster a more holistic understanding of complex issues.
Publication Accessibility
In the future, Semantic AI could play a crucial role in making academic publications more accessible. Translating dense technical language into summaries or translating content into different languages could democratize knowledge and break down barriers to information, both for the general public and for academics in lower-resourced regions or non-native English speakers.
Predictive and Prescriptive Analytics
Semantic AI’s ability to predict future trends could lead to a forward-looking academic culture. Institutions may start to rely on AI-driven insights to set research agendas, allocate funding, or build infrastructure for research on predicted future hot topics. This prescriptive approach could make academia more proactive rather than reactive, aligning research initiatives with future societal needs.
Enhancing Peer Review
Semantic AI could significantly reform the peer review process. By analyzing and comparing content for originality, methodological rigor, and relevance, these systems could provide initial screening for reviewers, ensuring that human effort is reserved for the most promising or innovative submissions. This could speed up the publication process and enhance the overall quality of accepted papers.
Impact on Learning and Teaching
Beyond research, Semantic AI could influence learning and teaching by offering personalized educational resources, reading materials, and interactive learning experiences based on the semantic understanding of a student’s strengths and weaknesses. This could lead to highly tailored educational experiences.
Ethical Considerations
As with all AI-powered tools, the use of Semantic AI in academia must be guided by ethical standards. Concerns about privacy, data security, and the fair use of AI will need to be addressed. Moreover, reliance on AI should not overshadow the value of human insight; instead, it should complement human intellect.
Economic and Societal Impact
If Semantic AI tools become widespread, the economic model for access to scholarly communication might shift. Subscription models could be replaced by more open-access content driven by a need for AI readability. Additionally, the societal impact of research could be evaluated based on a semantic understanding of a paper’s content, influencing funding and policy decisions.
In summary, integrating Semantic AI into academic publishing is expected to make research more efficient, cross-disciplinary work more feasible, academic insights more accessible, and the academic enterprise more ethically aligned with societal values. However, it will be important for academia to navigate these changes with caution to ensure that the quality and integrity of academic work are maintained alongside the adoption of these technological advancements.
While AI excels at processing vast amounts of data, human creativity and critical thinking remain vital to academic inquiry. Semantic AI is best leveraged as a collaborative tool to empower researchers. For example, machine learning algorithms can scan millions of academic articles to surface publications relevant to a researcher’s niche interests and questions. This expands the scope of what an individual scholar can explore, augmenting the reach of human curiosity.
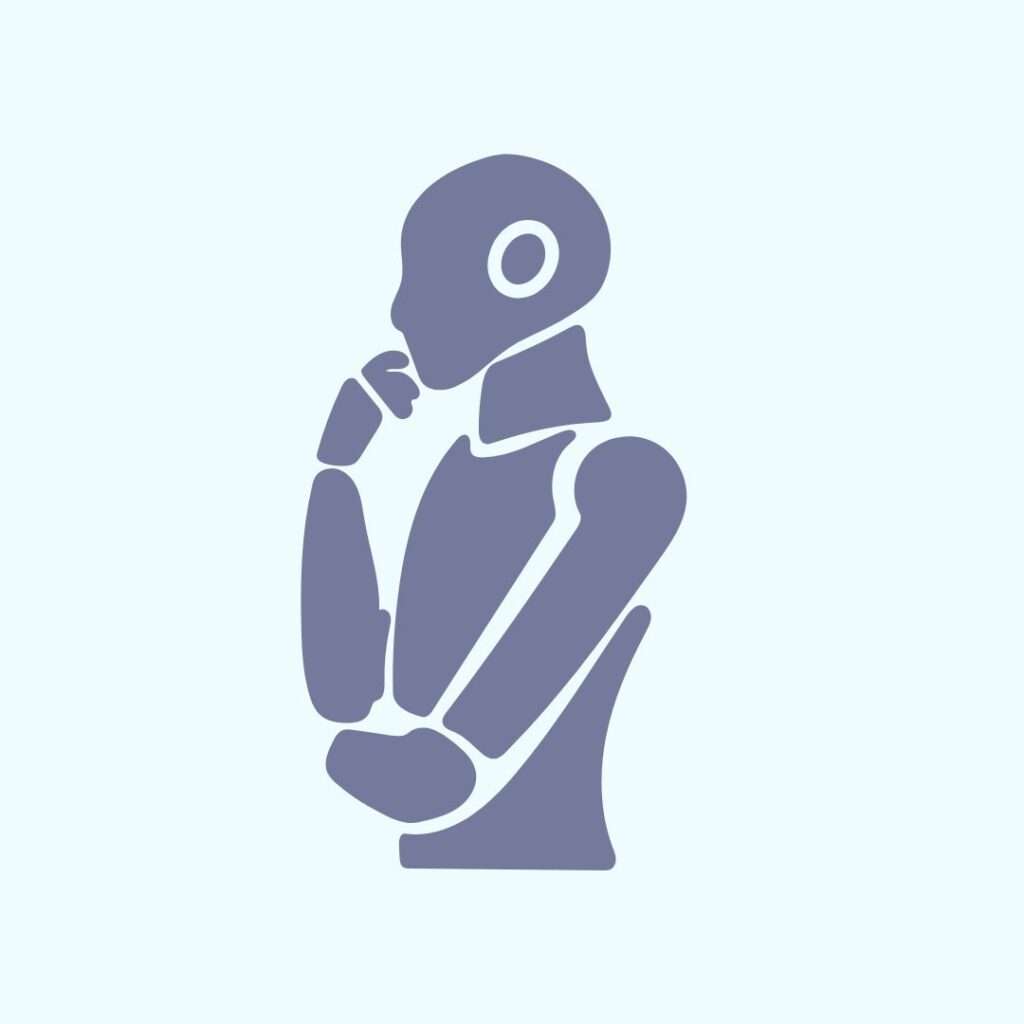
As with any new technology, implementing Semantic AI on a large scale within academia raises critical ethical questions. There are valid concerns about perpetuating societal biases, compromising personal privacy, and researchers becoming over-reliant on algorithms. Academia must establish governance policies and best practices for accountable, transparent, and ethical utilization of AI. Ongoing education on avoiding pitfalls like bias while harnessing benefits like increased access to knowledge will be essential.
Conclusion – Embracing the Intersection of Semantic AI in Academia
Integrating Semantic AI in academia and publishing offers numerous benefits, including improved efficiency, enhanced quality control, and expanded knowledge discovery. Researchers can uncover new insights and connections across disciplines by leveraging machine intelligence to process vast amounts of data and identify patterns. Additionally, AI tools can assist in screening submissions for plagiarism and errors, lightening the reviewers’ workload and allowing them to focus on higher-order assessments.
However, the adoption of Semantic AI also presents challenges and ethical considerations. Academia must establish governance policies and best practices to ensure fairness, transparency, and accountability in using AI. Concerns about bias, privacy, and over-reliance on algorithms must be addressed through ongoing education and responsible utilization of advanced technologies.
Ultimately, the future of academic research lies in the collaboration between human curiosity and machine intelligence. By embracing Semantic AI as a collaborative tool, researchers can harness its power to augment their capabilities and expand the boundaries of knowledge. With careful consideration and responsible implementation, Semantic AI has the potential to revolutionize the academic landscape and shape the future of research and publishing.