Table of Contents
- Introduction
- Understanding Generative AI: Unpacking the Meaning
- Applications of Generative AI
- Ethical and Social Implications of Generative AI
- Conclusion
Introduction
Generative AI refers to a class of AI systems capable of generating new, original content such as text, images, music, and more. Understanding generative AI allows us to explore its full potential and future uses. Unlike other types of AI focused on analysis or classification, generative AI models create novel outputs based on patterns learned from training data.
While most AI systems excel at tasks like identifying objects in images, translating languages, or making predictions and recommendations, generative AI models do the opposite—they create completely new artifacts like text, art, music, and more. The key difference lies in the ability of generative models to produce novel, creative content by learning the underlying structure of training data and using that to generate new examples.
Generative AI is enabling new forms of creativity and expression across many domains. In art, models like DALL-E 2 and Stable Diffusion can generate amazingly realistic and stylistically diverse images from text prompts. For music, AI systems like Jukebox and Magenta Studio can compose songs in different genres and instruments. In language, models like GPT-3 display human-like writing abilities for various applications.
This democratization of creativity has massive implications. Anyone with an idea can potentially bring it to life with AI. Generative models are also helping push the boundaries of what’s possible in their respective mediums.
Examples of popular generative AI models:
- DALL-E 2 and Stable Diffusion (image generation) – Enabling new forms of visual art and design
- GPT-4 (text generation) – Automating content writing and customer support interactions
- Jukebox and Magenta (music composition) – Assisting human composers and musicians
While concerns exist around potential misuse, generative AI has empowered people without great technical skills and access to cutting-edge algorithmic creativity. Responsible deployment will be key to maximizing societal benefit as the technology matures.
Understanding Generative AI: Unpacking the Meaning
Generative AI is a rapidly evolving field that promises to unlock new levels of creativity and innovation. But what exactly does it mean?
At its core, generative AI refers to systems that can create original content like images, videos, music, or text that are unique and often indistinguishable from human-made works.
Put simply, generative AI allows computers to generate new artifacts and content by learning from vast datasets. The key capabilities that set generative AI apart include:
- Learning patterns and structures from training data sets of images, text, audio, etc.
- Leveraging that learning to produce new, original output that resembles human-created work.
- Continuously improving output quality based on feedback to become increasingly skilled at generation tasks.
So, in essence, generative AI takes in data, identifies meaningful patterns, and uses those learnings to create something novel. The more data it trains on, the better it gets at independent generation within a domain.
Several key innovations in machine learning and neural networks over the past decade have given rise to the current explosion of generative AI capabilities:
- Advances in deep learning and neural networks – Complex neural net architectures can now learn robust generative models from data at scale.
- Rise of generative adversarial networks (GANs) – GANs pit two neural nets against each other to generate increasingly realistic synthetic data.
- Transformers and foundation models – Models like GPT-4 (and the subsequent updates) demonstrate an ability to generate remarkably coherent text by learning patterns from vast text corpora.
- Diffusion models – By adding controlled noise, diffusion models can reshape noise to yield realistic images and audio generation.
These and other breakthroughs have brought about unprecedented creative potential at the intersection of AI and machine creativity.
The applications of generative AI span far and wide, including:
- Automating rote design and content creation tasks to augment human creativity
- Enabling rapid ideation and concept prototype development to accelerate innovation
- Producing creative assets like logos, images, and music that can inspire new directions
- Personalizing content by generating tailored recommendations and custom creations
As generative models advance, they promise to democratize access to beneficial AI while reducing economic barriers to creativity and innovation. Responsible development of these technologies may profoundly transform entire industries in the years ahead.
Applications of Generative AI
Generative AI is finding real-world applications across a wide range of creative fields. In content creation, generative models can assist human writers by suggesting ideas or generating full articles on a topic. AI writing tools like Jasper and Sudowrite leverage large language models to transform outlines into long-form content. Though quality control is still needed, this can significantly boost content productivity.
In graphic design and product development, generative AI allows designers to instantly create, iterate, and select from hundreds of designs. For example, platforms like Anthropic generate product images to train e-commerce algorithms or help designers visualize. Generative design can also automate early-stage ideation to focus creative efforts where humans still excel—assessing quality, art direction, and style.
Examining Ethical Considerations
Despite promising applications, employing generative AI in creative pursuits raises some ethical questions. As these models produce original designs, stories, and art, they introduce complex issues around copyright, ownership, and authenticity. If an AI generates a product design, song, or meme, who owns it? How do we balance public access with protecting individuals’ intellectual property?
There are also concerns that over-reliance on generative tools may discourage human creativity, critical thinking, and skill-building. Responsible integration requires nurturing human talent while leveraging AI for efficiency—a delicate balance. Policymakers, researchers, and practitioners must collaborate to develop ethical frameworks as generative AI permeates creative sectors.
Showcasing Success Stories
While considering ethics, we must highlight cases where organizations have positively embraced generative AI.
For example, graphic design marketplace DesignBro uses AI to offer small businesses high-quality logo designs at affordable prices. This expands access to critical branding services. Educational publisher Pearson leverages AI co-writers to increase content production for personalized learning programs at scale. Both show how, when thoughtfully implemented, generative AI can extend human capabilities to more people.
As the technology matures, more human-AI creative collaboration will likely emerge. But this requires discussing both profound potential and ethical challenges to develop equitable, responsible policies enabling generative AI for social good.
Ethical and Social Implications of Generative AI
As generative AI advances, concerns around intellectual property rights and the authenticity of AI-generated content have emerged. Who owns the copyright to art, music, or text created by an AI system? How can we verify the originality of generative content? Critical questions need thoughtful solutions as generative AI becomes more ubiquitous.
Several strategies could help address these concerns:
- Developing watermarking or signature techniques that tag AI-generated content
- Creating registries that record the lineage of generative models
- Establishing standards around transparency and disclosure when publishing AI-generated content
- Rethinking and updating intellectual property policies to account for creative works by AI systems
By proactively tackling issues around rights and authenticity, we can realize the creative potential of generative AI responsibly.
The rise of capable generative AI systems sparks important questions about how society may be impacted:
- What job roles might become less relevant due to automation by generative AI?
- Could the democratization of content creation excessively flood the market?
- How could over-reliance on AI creativity dull human imagination and innovation?
While definitive answers remain elusive, continuously evaluating and discussing these concerns is vital. Responsible policies and strategies anticipating the societal effects of advancing generative AI can help smooth the transition rather than reactively deal with consequences.
Some best practices for deploying generative AI include:
- Prioritizing transparency, auditability, and accountability
- Developing testing protocols focused on safety and ethics
- Creating oversight processes involving diverse perspectives
- Investing in research on AI ethics and responsible innovation
Adopting an interdisciplinary, collaborative approach that brings together technology leaders, policymakers, domain experts from impacted fields, ethicists, and civil society advocates can enable constructive dialogue and responsible progress.
Proactively self-regulating and participating in wider public discourse, the generative AI community can lead the responsible path forward amidst rapid technological leaps.
Conclusion
This discussion on generative AI has highlighted several key takeaways. First, generative AI represents an exciting new frontier in technology with immense creative potential. However, as with any powerful technology, it also carries risks if deployed irresponsibly. When leveraging generative AI, organizations must make ethical considerations around intellectual property, authenticity, and societal impact.
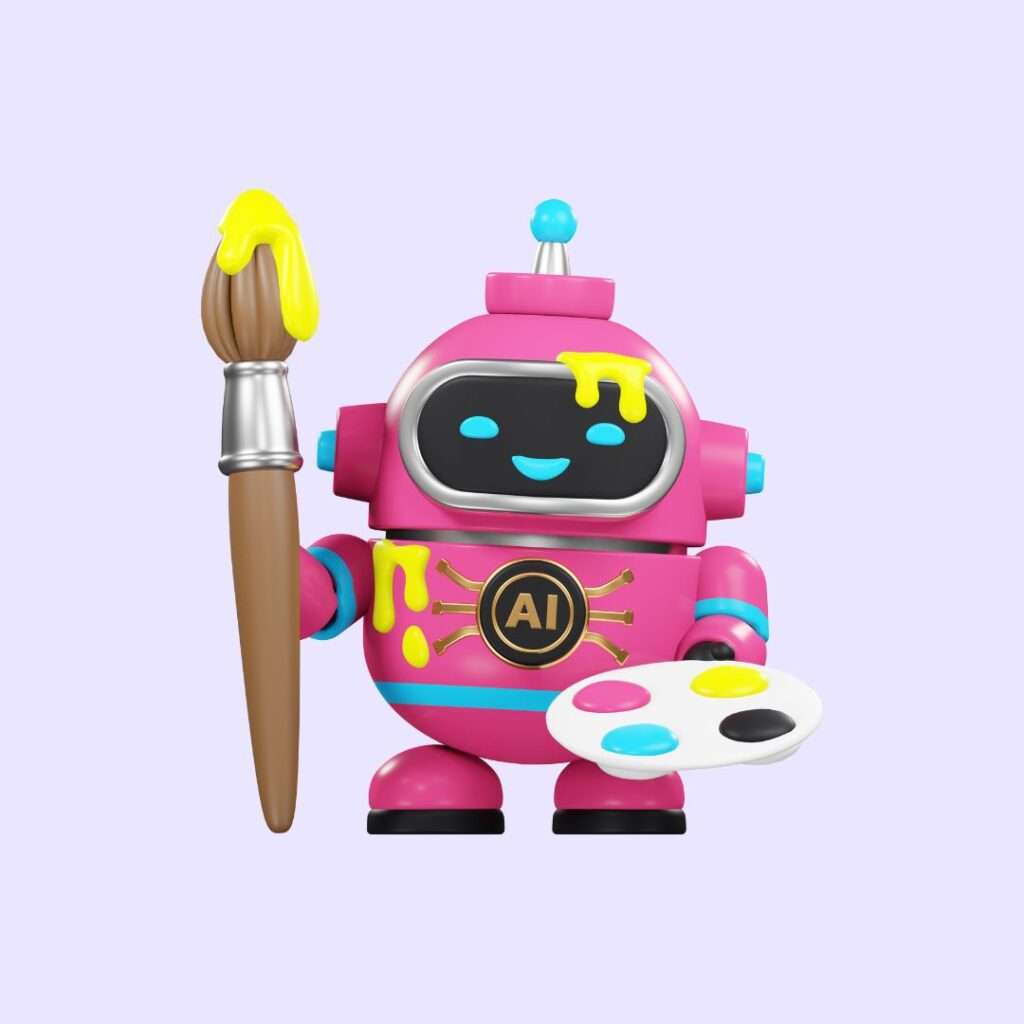
A nuanced, well-informed public dialogue around these technologies will be critical as we advance. All stakeholders—including tech companies, policymakers, and civil society—have a role in shaping the responsible development of generative AI. As citizens, we must educate ourselves on the benefits and challenges to make wise choices regarding whether and how these technologies are applied.
The key takeaways from this discussion are:
- Generative AI allows computers to create original content and art by learning from vast datasets.
- It has promising applications in content creation, design, story generation, and many other creative fields.
- Generative AI has the potential to automate certain job roles, making them less relevant in the future. This could include tasks such as content creation, design, and even some aspects of storytelling.
- The democratization of content creation through generative AI could lead to an excessive flood of content in the market. This could make it harder for individual creators to stand out and find success.
- Over-reliance on AI creativity could potentially dull human imagination and innovation. If humans rely too heavily on AI systems to generate ideas and create content, they may become less inclined to think creatively themselves.
To ensure responsible deployment of generative AI, transparency, auditability, and accountability should be prioritized. Organizations should also develop testing protocols focused on safety and ethics and create oversight processes that involve diverse perspectives.
Investing in research on AI ethics and responsible innovation is crucial. It is important to understand the potential societal effects of advancing generative AI and to anticipate and address any negative consequences.
Adopting an interdisciplinary and collaborative approach that includes technology leaders, policymakers, domain experts, ethicists, and civil society advocates can enable constructive dialogue and responsible progress.
The generative AI community should proactively self-regulate and participate in wider public discourse. By leading the responsible development of generative AI, they can help positively shape its future.
In conclusion, generative AI has great potential but must be deployed responsibly. Ethical considerations, transparency, and collaboration are key to ensuring the benefits of generative AI while minimizing any negative impacts. It is important for all stakeholders to educate themselves and actively participate in shaping the future of this technology.
1 thought on “Understanding Generative AI”